Industrial engineers are turning to AI to build advanced process simulation solutions and accelerate progress toward fully autonomous operations in the energy, power, and chemicals industries.
Deep reinforcement learning (DRL) agents, infused with AI, are helping transition traditional industrial automation to industrial autonomy for core control, field, and production processes. This use of agentic AI for advanced reasoning and iterative planning with human-in-the-loop aims to enable new applications in advanced industrial control, system-level autonomy, and fully autonomous plants.
In this post, we explore how AVEVA, a leader in industrial software, integrated its Dynamic Simulation platform with the NVIDIA Raptor DRL engine to maximize industrial plant production, reduce unplanned downtime, and enhance product quality.
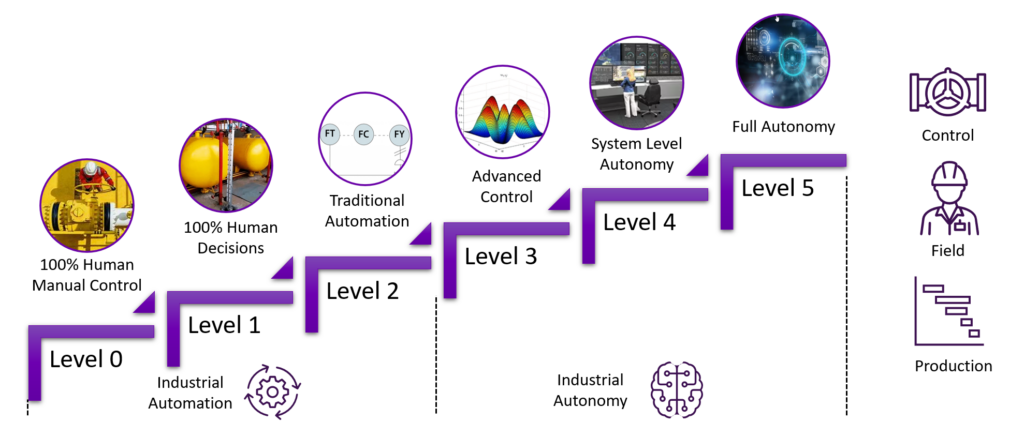
Challenges and benefits of industrial automation
Conventional advanced control technologies, such as advanced process control or closed-loop real-time optimization are best-fit for repeatable processes. This occurs when plant conditions are relatively constant to optimize energy consumption, emissions, and production. However, industrial plants undergo transient events, such as imposed feed changes or unexpected disturbances, which are difficult to control using either base level or advanced process controls.
In these dynamic situations, industrial plant operators switch to manual control to re-stabilize the plant and avoid unwanted and expensive consequences, such as a power plant trip when a power-generating turbine is automatically shut down due to an unexpected event.
By achieving greater industrial autonomy, plant operators, managers, and engineers can achieve several benefits:
- Enhanced safety: Reduces worker exposure to hazardous environments.
- Increased operational efficiency: Optimizes production and equipment use.
- Cost reduction: Optimizes labor and maintenance costs.
- Improved decision-making: AI-driven insights for better accuracy and performance.
- Environmental sustainability: Optimizes resource usage and reduces emissions.
- Scalability: Enables efficient management of large or remote operations.
- Connected remote operations: Centralized control of geographically dispersed assets.
Building an industrial automation strategy
AVEVA and Schneider Electric are bringing their industrial expertise to collaborate with NVIDIA across industrial process, hybrid and compute infrastructure use cases:
- Autonomous AI agents trained using physically accurate industrial digital process twins at scale on a high-speed compute architecture.
- High-fidelity digital twins at scale for industrial 3D environments and unified operation centers to monitor and control industrial operations in real time.
- Data center reference designs for NVIDIA-accelerated compute clusters that enable operators to support AI workloads on their data center infrastructure, improving the speed of deployment and reliable life-cycle operation.
Results of AVEVA Dynamic Simulation with NVIDIA Raptor
AVEVA Dynamic Simulation, a rigorous first-principles simulator for industrial processes, was tested using an integration with NVIDIA Raptor, a deep reinforcement learning engine.
AVEVA Dynamic Simulation is used to build dynamic simulation twins of process plants for operator training simulators, control checkouts, and process design across multiple use cases.
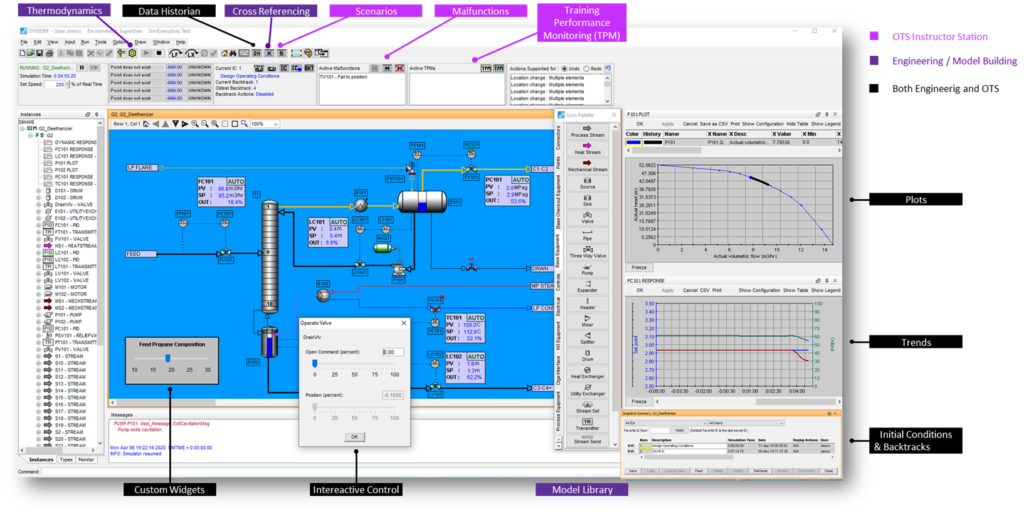
Industrial engineers use AVEVA Dynamic Simulation to reduce operating costs, speed time to market for industrial products, enhance worker safety, and optimize plant uptime across a variety of heavy industries.
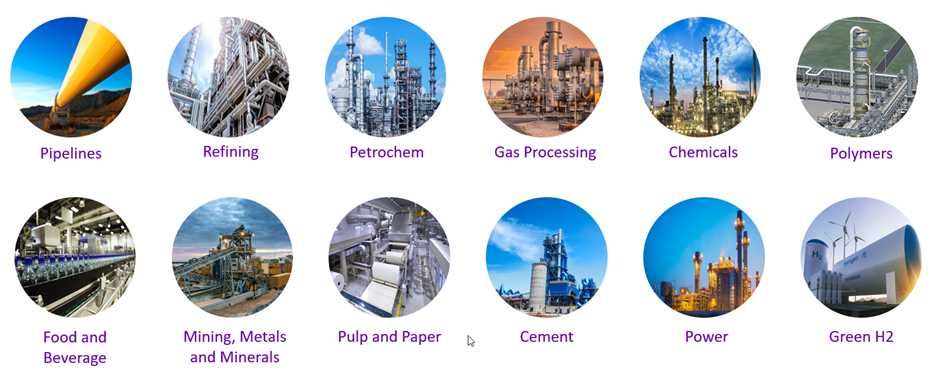
Raptor was trained offline using AVEVA’s dynamic process simulation of industrial process plants. Raptor enables large-scale reinforcement learning training using accelerated computing on-premises or in the cloud.
NVIDIA used the Raptor framework internally for chip design using reinforcement learning. Using Raptor, NVIDIA generated arithmetic circuits with a 25% smaller footprint, while maintaining similar performance and accuracy to leading electronic design automation software solutions. Because Raptor supports a wide range of reinforcement learning algorithms, such as proximal policy optimization, it can be put to use to solve industrial problems such as AVEVA Dynamic Simulation.
The AVEVA Dynamic Simulation model environment takes an action from the NVIDIA Raptor deep reinforcement learning agent, outputting a new state to Raptor. At each step, the DRL agent gets a reward depending on whether its action resulted in a better or worse state. Raptor learns by maximizing rewards over a series of actions.
When trained, the resulting control policy can be deployed in the plant control system:
- Minimize production impact of plant upset by stabilizing product quality in a shorter time than a manual plant operator.
- Improve control of batch processes to deliver consistent and repeatable product quality.
- Reduce complex startup and shutdown times to maximize production throughput.
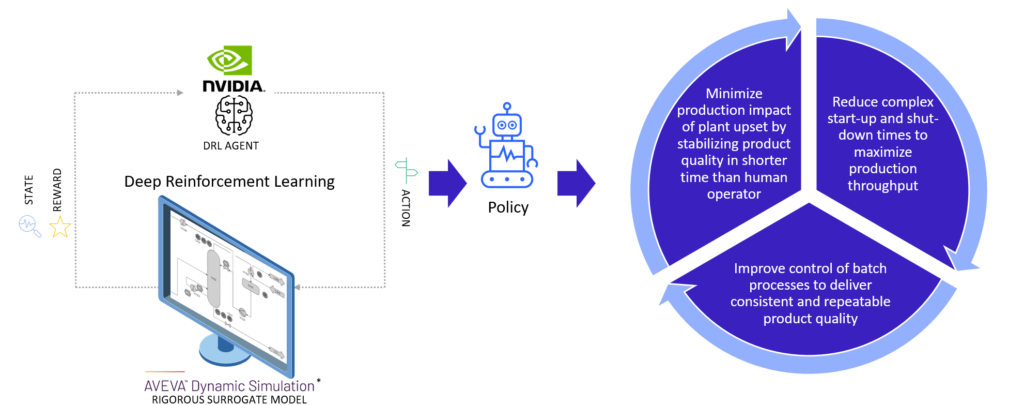
An example industrial problem is a chemical distillation process to separate heavier propane from lighter methane and ethane. When the incoming feed changes by a larger-than-expected amount, the industrial process is disturbed and conventional controls might not manage levels and product qualities in the correct ranges. Normally, this would require plant operator intervention.
A Raptor DRL agent was trained using AVEVA Dynamic Simulation to control heat input to the column, column bottoms level, and reflux drum level. As a result, the DRL agent could stabilize the distillation system twice as fast as a manual plant operator with temperature and product quality remaining in the correct ranges even during large changes in feed, in contrast to conventional industrial base controls.
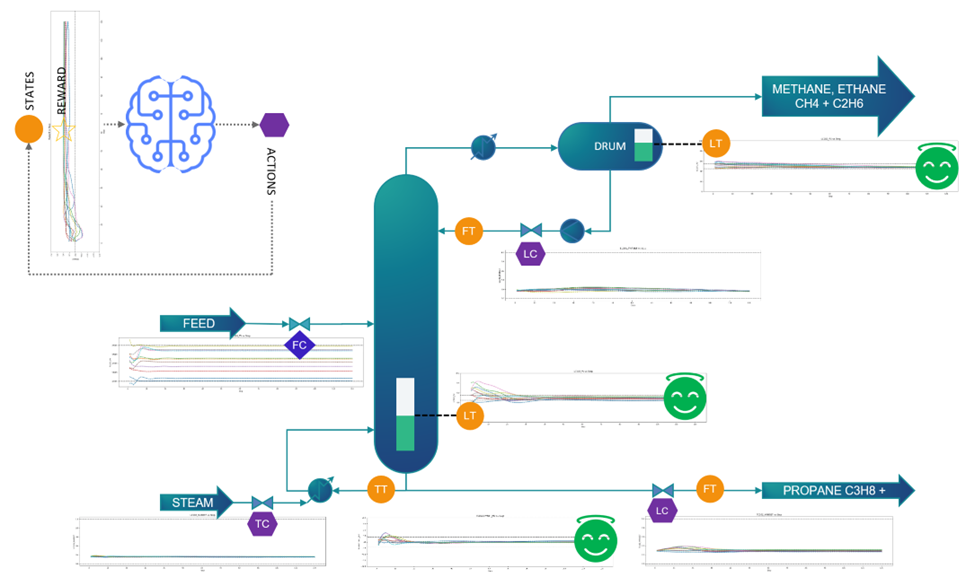
Future of industrial-process simulation
AI and deep reinforcement learning are enabling industrial plant process control to achieve greater levels of autonomy. AVEVA’s accurate process digital twins and the NVIDIA-accelerated computing platform are driving innovation in industrial process simulation from proof of concept to real-world deployments.
The next steps for AVEVA are to pilot, productize, and scale AVEVA Dynamic Simulation with Raptor. AVEVA plans to work with some of the world’s biggest energy companies to pilot the solution—an essential first step for customers to gain confidence. A key advantage is that AVEVA Dynamic Simulation with Raptor can be trained, tested, and proven entirely off-line from the actual industrial power plant.
After customer pilots have successfully been completed, AVEVA will look to productize and scale up the solution using no-code workflows in AVEVA Connect. This will enable faster training using the NVIDIA-accelerated computing platform with plug-and-play modules for testing and deployment of deep reinforcement learning agents.
Learn more about the AVEVA and NVIDIA collaboration in industrial process simulation. Explore AVEVA Dynamic Simulation and NVIDIA Raptor.